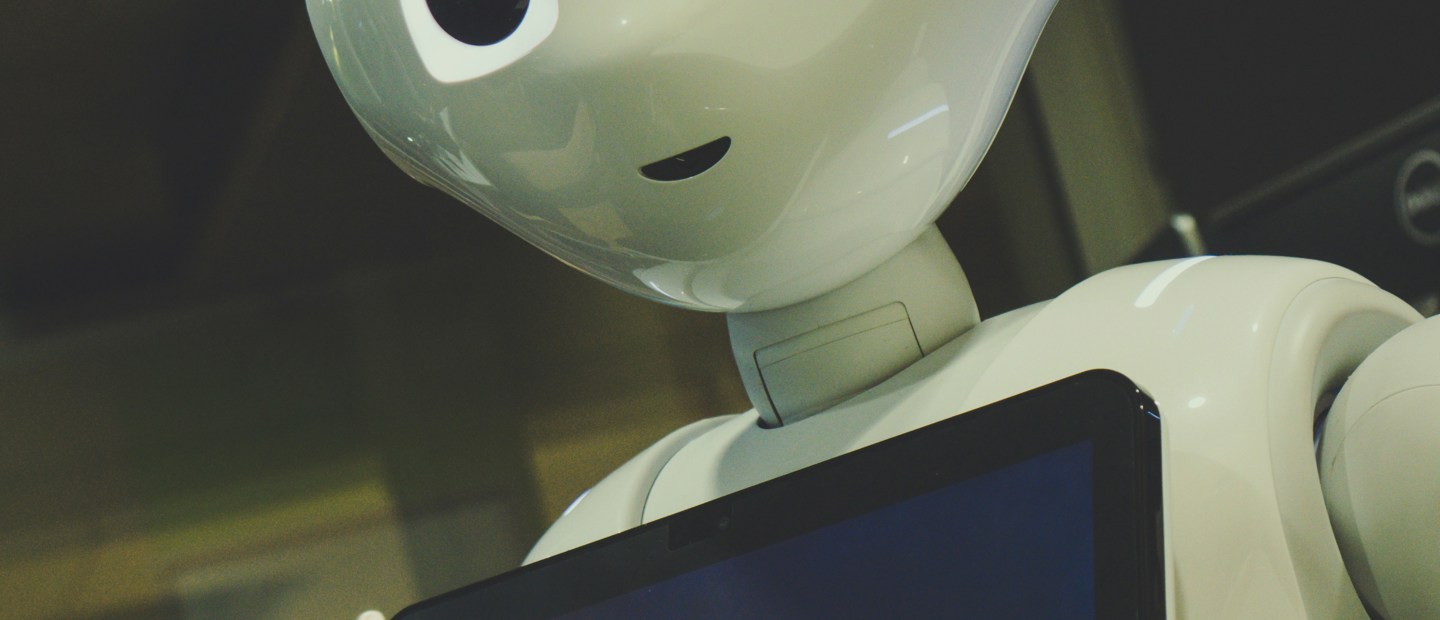
As AI enters the negotiation room, it's critical to understand its impact and how to adapt. Will AI lack human touch or bring unprecedented efficiency? The future of deal-making is evolving and so must negotiators.
Imagine you are a key account manager (KAM) for a global tool manufacturer, and you have been doing business with a large national retailer for two decades. Both companies have profited from this relationship over the years and view the partnership as sustainable and important to their business models. One day, when it is time to start joint business planning (JBP) between these two companies, the retailer announces a change in policy. No more human contact for negotiations and all vendors will be negotiating with an AI bot before speaking to anyone.
How will this policy change impact the relationship, profitability and sustainability of both businesses? No one knows yet but negotiating using AI sounds intriguing, cutting edge and could reduce expenses, so several large retailers have been adopting AI for their negotiation with mixed results.
AI negotiation frameworks are not new and artificial agents have existed for over 25 years. Previously referred to as “automated agents” in British Telecom’s ADEPT project (Advanced Decision Environment for Process Tasks) in the 90s, the autonomous agents interacted with each other to create complex price quotes for customers and negotiate contracts between BT and their suppliers. In a published study by Kim Binmore and Nir Vulkan in 1999, the team used AI constructs to create independent negotiating agents. The constructs were among the first to combine game theory and microeconomic theory to create autonomous negotiating agents.
The models were run for millions of iterations and quite frankly the automated negotiation agent’s results were lacklustre. The team discovered that in negotiations with preexisting relationships or more complex negotiations, artificial agents became competitive rather than collaborative. The AI negotiations increasingly ended in stalemate, similar to the ‘tic-tac-toe’ simulations from the movie “War Games”. Regardless of how each agent performed its plotted proposals through multiple iterations, the agents became competitive. Difficult to say, but this might have been the first computer ‘ego’ malfunction.
John Nash laid the groundwork for general non-cooperative and cooperative bargaining theory in the 40s and 50s at Princeton (Economic Sciences, p.161). However, his equilibrium concepts were only picked up by economists decades later because his mathematical models were too complex to use with microeconomic modeling at that time. Our current state electronic computers are now equipped with the processing speed to reduce AI query response time and with the advent of qubit quantum computing, processing cycles will no longer be an issue in the future.
Some start-up enterprises are yet again combining game theory and microeconomic theory to create autonomous negotiating agents reminiscent of the 1990s. However, this time the AI negotiation models are based on a neural network that already contains multiple negotiation scenarios, as well as a Large Language Model (LLM) database to serve as guidance for proposals. Two different types of models are currently in use for AI, the GPT model and the LLM model. The key difference is that the GPT (as in ChatGPT) is developed to compile large amounts of data and provide an output (based on the particular GPT’s purpose) in response to user requests and prompts. Building on the GPT framework, the LLM model covers larger categories of language models and scenarios (including GPT models). Only time will tell if the agents will find themselves in situations where they have an incentive to lie, act tough or exploit other strategic avenues not usually associated with machines, as discovered in the 1990’s (Binmore & Vulcan, p.1).
Who is using AI for negotiations today and why?
A November 2022 HBR article titled, ‘How Walmart Automated Supplier Negotiations’ tells the tale of how Walmart created boilerplate negotiation terms with over 20,000 of its smaller vendors for indirect procurement items (items not for resale to consumers). The HBR team noted that “the cost of hiring more human buyers to negotiate with them would exceed any additional value”. The indirect market is analogous to the stock market with raw material pricing set via indices and the calculation for an indirect purchase (such as paper towels for Wal-Mart’s bathrooms) is based on the raw material inputs, a labor component, and any other variables.
Only one-sided results were lauded by Walmart and HBR, with no independent reporting validating or disputing assertions that the new system saved money. So, the question is what additional ROI (other than eliminating new procurement jobs) was obtained? Furthermore, both the effectiveness of the negotiations conducted and the impact that a transactional bot had on interpersonal relationships were never quantified or shared. They must ask themselves whether they would prefer to have negotiated rock bottom costs or if they preferred damaging existing vendor relationships (and possibly destroying a vendor’s financial stability and its ability to service the Walmart business).
In late 2023, a CNBC article by Ryan Browne touted an AI negotiation between two bots as ‘a world first’ AI negotiation with no human involvement. The only human element was simply signing the resulting agreement. The London based AI firm, Luminance, simulated its Autopilot program and navigated its LLM and legal documents to analyze legal clauses, red line, craft new clauses and finalize the contract for a human signature. While this Autopilot acted as a ‘legal co-pilot’, both simulated legal teams were working based on a pre-negotiated agreement parameter set by humans. The Autopilot’s primary role was to eliminate much of the time-consuming legal work required to finalize a legal agreement, rather than negotiating the commercial aspects of the deal before the legal documents. AI that eliminates rote and commonplace repeated tasks can provide value but still requires human oversight.
Earlier this year, Wharton produced a negotiation practice GPT based on OpenAI’s ChatGPT bot framework. One of my colleagues decided to outline a negotiation scenario to determine how the bot would respond. After pulling back the veil of bot LLM information, it appears that the bot was fed on negotiation cases from the Harvard Business School. In this instance, a scenario was posed to the bot that outlined the variables in the price increase negotiation between my colleague, the hypothetical seller of chilled foods and the buyer, a large retailer.
The output was as my colleague called it ‘a bit soft’ since the bot assumed that the retailer would be highly collaborative, whereas price increase actions can frequently bring out a retailer’s competitive side. The bot did provide an agenda for the meeting based on the input variables but did not allow for a real ‘mock negotiation’. Instead, the bot outlined both parties’ responses and then asked the user if any changes were required. Even when the bot was told that the retailer was being competitive, the bot continued to use a highly collaborative negotiation style. At a simplistic level, this is a bot that gives an overview of how a negotiation might play out but would not prepare someone for a dynamic, high-pressured live negotiation.
While AI can be a valuable tool for preparing, recording and reviewing negotiation results, it currently has limitations in a negotiation environment. The primary challenge is that AI does not have the ability to dynamically create value during a negotiation, which can lead to a more transactional and competitive approach. Creative and adaptive adjustments during negotiation are sometimes warranted, and AI is not currently equipped to receive the necessary feedback to adjust its negotiation approach.
Additionally, the dynamics of emotion cannot be properly assessed during a stressful negotiation to fine-tune the estimate of the other party’s breakpoint during the heat of the deal. Furthermore, AI does not know how to use emotional intelligence to determine when to take a ‘time-out’ to allow cooler heads to prevail during a negotiation and will most likely be deadlock in these instances. Although AI is continuously evolving its LLMs based on inputs and new content on the web, it is only as good as its inputs and its experience with requests and handling data.
One challenge on the horizon is AI’s voracious need for new, readily available data to build and evolve LLMs. Since most of the proposals and counterproposals in negotiations are not digitized and commercially shared, AI can only work with a limited and sterile data set of case study negotiations when formulating proposals. In the AI realm, there is a data paradox unfolding that could have catastrophic future implications. Phillip Russom of TDWI commented in 2021 that, “to broaden and enrich the correlations made by the [AI build] algorithm, machine learning needs data from diverse sources, in diverse formats, about diverse business processes.”
When AI simulations ran out of new data, the AI system started teaching itself on data that it had created. This is called a ‘fully synthetic learning loop’. When AI had a deficit of newly created data from humans, researchers found that these fully synthetic loops inevitably lead to Model Autophagy Disorder (MAD) with either the quality (precision) or diversity (recall) of the generated text and/or images progressively decreased over successive generations. To prevent the AI model from going MAD, a fresh influx of human-generated data is required.
The majority of the content being created daily on the web consists of AI bots generating images, articles and other content types. While this saved a lot of copywriters’ and reporters’ time, it left a ballooning deficit of newly created human content. To determine which online content is synthetic or organic, prominent figures in the AI industry made commitments at the White House to introduce strategies such as watermarking for distinguishing synthetic data from authentic data. Although this approach is in its infancy and voluntary, hopefully, it will serve as a foundation of metadata to categorize online content. Only time will tell if any AI LLMs cause a bot to go MAD, but humans need to keep creating new content to keep feeding the AI data beast.
In a 2021 Forbes article, Matt Przybyla, author and a senior data scientist, pointed out that, “there often still needs to be trained human guidance to AI and machine learning initiatives, especially if the output is critical to the tasks at hand”. Based on the current information of the AI industry regarding the negotiation, the AI application catchphrase from Bain’s recent AI report is, “If 2023 was about experimentation, 2024 is all about results” and this concisely sums up most CEOs perspectives regarding current and future internal AI financing initiatives.
Large organizations initially prototyped AI ‘off the shelf’ solutions to meet their needs, such as creating marketing content based on ChatGPT prompts, synthesizing data for a Data Warehouse, using AI to summarize documents and other generic tasks. However, because data quality and accuracy can be mission-critical, organizations are customizing internal AI solutions to fit their needs, so that they can own the data, the data quality, and the intellectual property (LLMs).
According to Bain, “In early 2024, 87% of companies they surveyed were developing, piloting, or have deployed generative AI” with commercial application in “software code development, customer service, marketing, sales and product differentiation” (i.e. product innovation). Bain makes a broad statement with a high percentage but notice that the spectrum of work and adoption range they cite is large. For example, of the 87%, who are deploying generative AI? The introduction of detailed implementation information would separate speculation from application but might detract from the impact of a company’s, ‘Hey, we’re doing AI!’ press release on their stock price. While executive leadership teams are agreeing to invest in generative AI, they should also consider the ‘human variable’ for internal alignment and adoption of their new practices. Without internal alignment before development, the predicted internal ROI will fall short of expectations.
When it comes to AI, and specifically in the negotiation of multimillion-dollar commercial contracts, the human variable cannot be underestimated. AI will add value to the preparation and planning phase to quickly consolidate large disparate amounts of data, but a person is required to assess the other party’s perceived power, their counterparts’ emotional proclivities and team dynamics. Additionally, a person is essential to building trust between two companies before starting a commercial relationship. Without the human variable, negotiations can either be collaborative, or competitive, but not both. The human component can sense changes in real time and adjust accordingly, rather than simply reacting to an AI proposal with numbers.
Long-term commercial relationships are built through person-to-person negotiations. By understanding the other party you are negotiating with, humans can ‘get inside the other person’s head’ to create value between large global companies. A negotiation culture framework can elevate a company’s internal dialogue and ensure that everyone is speaking the same commercial negotiation language and maximizing every negotiation opportunity.
Currently, AI is unable to create value through negotiating because it is unable to introduce uncommon creative variables into the negotiation dialogue, especially in unique large negotiations that are built on trust and where the contract’s value is high. However, it can be a great analytical, recommendation, and summary tool. For example, AI is good at providing prompts, assessing market conditions and generating insights to spark human creativity, but to obtain value for your company over time, you need to understand the other party and what they value.
To achieve your organization’s objectives, maximize your negotiations to show that you are adding to the company’s net profit, and all of this must be done faster than ever. There are many tools available to save time in mundane tasks so that you can focus on your psychological and emotional negotiation planning. Use your time wisely with AI, and you will save time planning for your next negotiation, but you also need to prepare for any curveballs that might be sent your way from the other party. The decision on whether you should implement an AI solution is up to you but choose wisely and don’t work on auto-pilot. Which is better, the red pill or the blue pill?
References:
CPG Growth beyond trade
https://www.bain.com/insights/future-of-grocery-easing-the-profit-squeeze-infographic/
Applying game theory to automated negotiation - Ken Binmore and Nir VulkanNetnomics 1 (1999) pages 1-9.The ESRC Centre for Economic Learning and Social Evolution, University College London.
How Walmart automated supplier negotiations
https://hbr.org/2022/11/how-walmart-automated-supplier-negotiations
What happens when we train AI on AI data?
https://insideainews.com/2024/04/19/what-happens-when-we-train-ai-on-ai-generated-data/#:~:text=Researchers%20found%20that%20these%20fully,progressively%20decreasing%20over%20successive%20generations.
How much people trust AI, and where?
https://www.bain.com/insights/where-the-us-is-most-ready-to-welcome-generative-ai-snap-chart/
About the author
Dr. Brian Denning, Ph.D., is an experienced negotiation professional with a background in sales, marketing and strategy, supported by an MBA, a PhD in Leadership and a PhD in Organizational Management. Brian has over 20 years of business experience, including managing multi-billion dollar P&L responsibilities, creating profit for both large and small companies and founding two start-ups from inception to profitability. His industry expertise spans hydrogen generation, automotive repair, building materials, CPG, textiles, technology, marketing, transportation, software and financial management. He specializes in large commercial negotiations, creative marketing and sales solutions and product portfolio management.
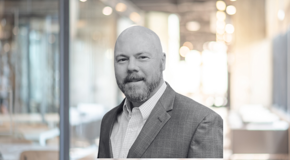
Subscribe to our newsletter
We’d love to send you our monthly round up of news, events and products.